

Overview
Summary
Practicum and Externship project partnered with Salesforce.
Team
4 UX Designers
1 Data Scientist
2 UX + 1 Eng. Leads @Salesforce
My Role
UX Research / Design
Duration
Sep - Dec 2022
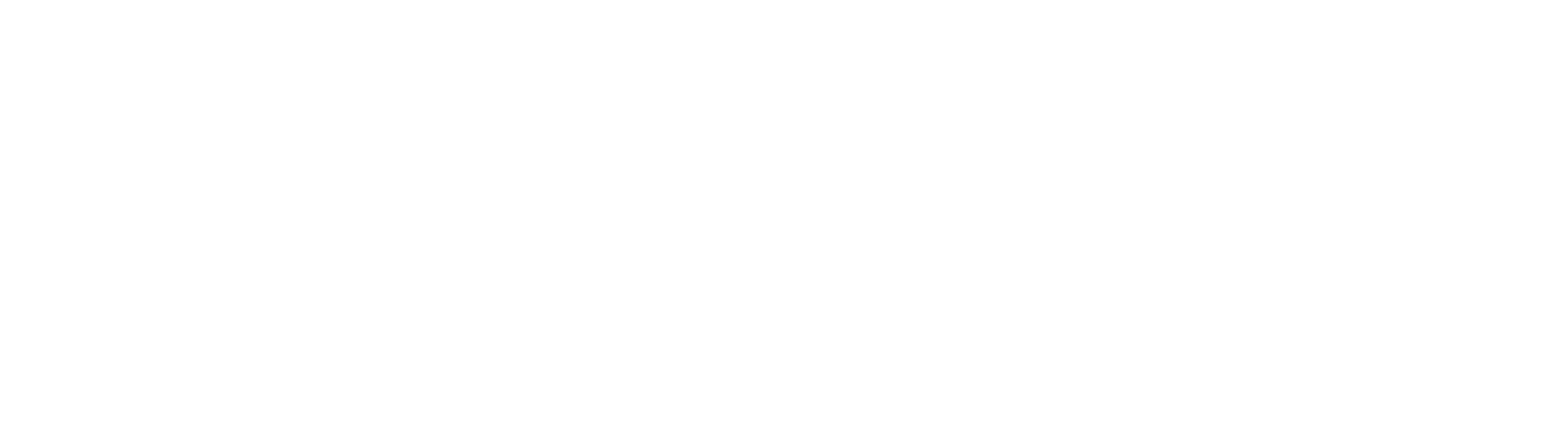
Background
Context
Einstein Prediction Builder (EPB) is a no-code AI prediction tool for CRM that allows customers to predict future business outcomes based on their own data without coding.
EPB: a no-code AI prediction tool for CRM
Problem
The adoption rate to EPB is low.
Salesforce team came to us with multiple problem statements. Adoption was low, tasks were left incomplete and models created with Einstein Prediction Builder were often not deployed. We needed to identify how to make it simply serve it's users.
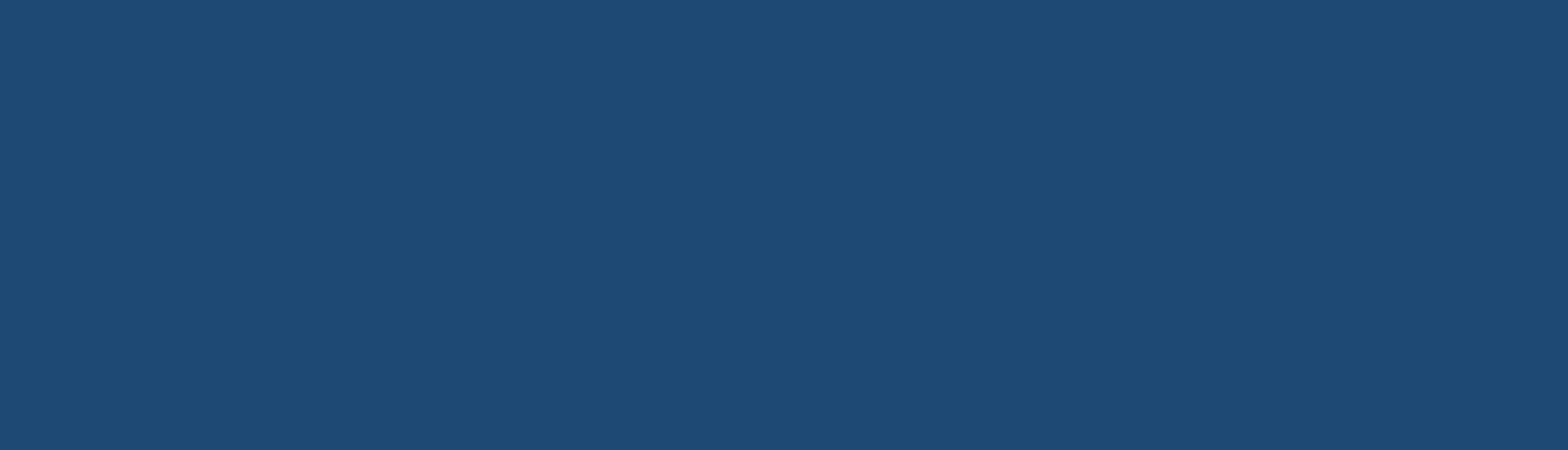
Why are users stoping using EPB?

Dig into the problem
Secondary Research
Understand the context through existing research
Due to the confidential nature of Salesforce's products, we were unable to conduct primary research for this project, so we learned from the extensive analysis from Salesforce and conducted heuristic evaluation ourselves to understand the features and pain points of existing users and products.
The REAL problem
The adoption rate is low
Business Ops aren’t confident to build their model in EPB
From research we found that the real problem behind it is that EPB’s target users, Business Operations Interpreter, stop using EPB as they are not confident about the results of the model. As low AI maturity users, they often get stuck in a series of steps without understanding its purpose in the prediction process.
Opportunity
Balancing simplicity and empowerment
After further summarizing the existing problems and current market offerings, we found that different levels of user groups require different levels of understanding of AI knowledge, and thus different levels of interface complexity. Starting from the needs of our target users, we found that the opportunity lies in balancing Simplicity and empowerment: streamlining user experience while enhancing AI Model understanding.
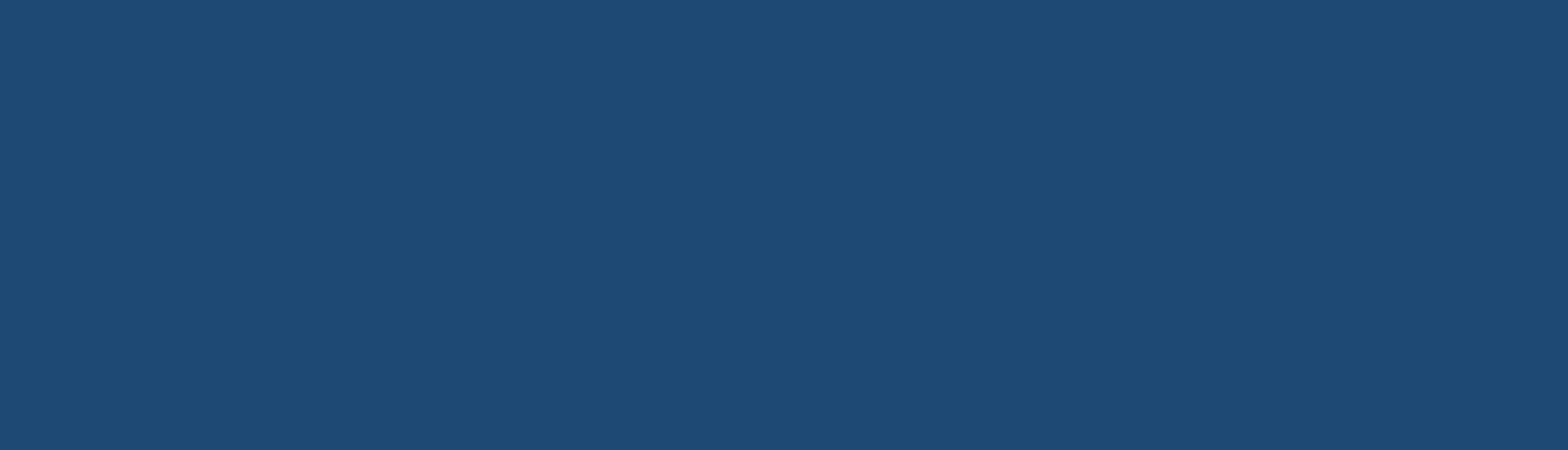
How Might We...
Help users ease the burden of each step
while enhancing overall understanding of the AI model,
thus empowering them to generate business insights?
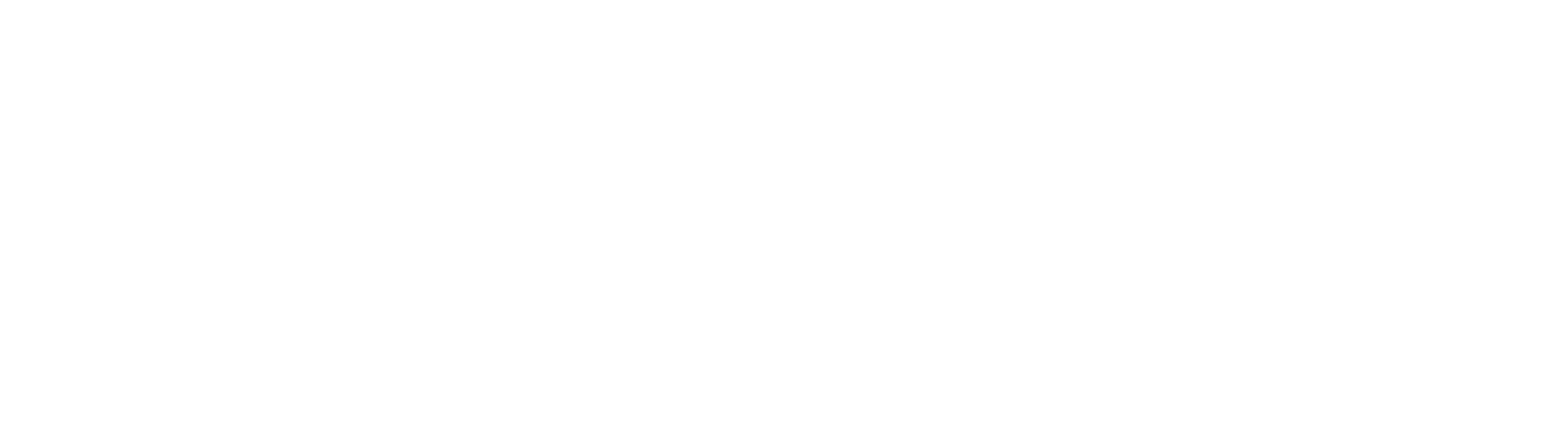
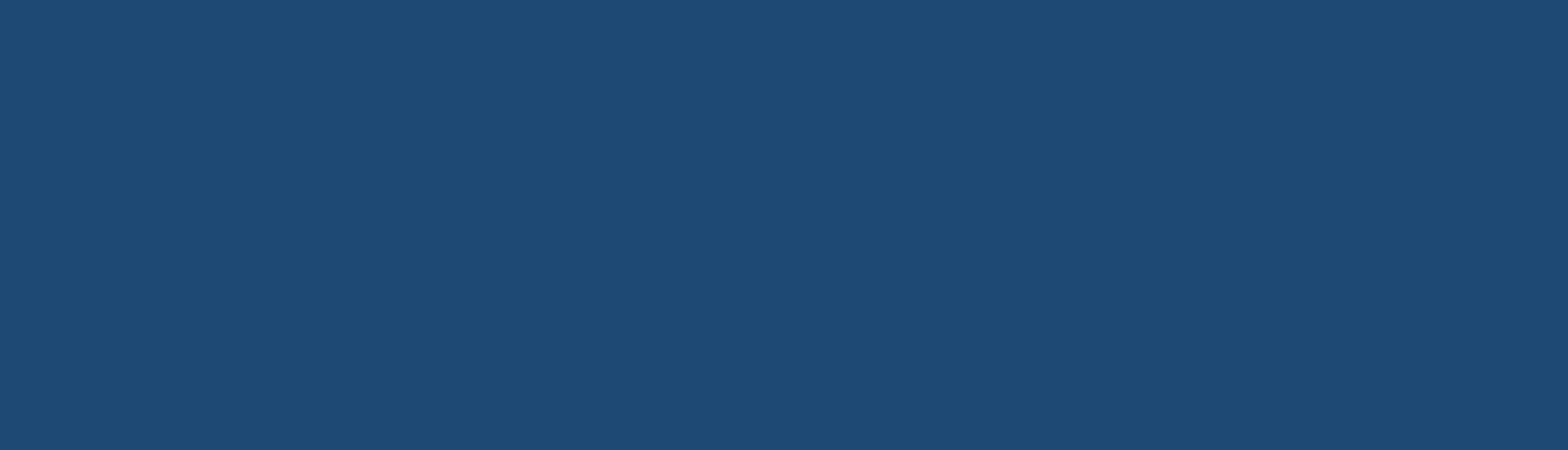
Design Decisions
What to consider when designing for a complex AI enterprise tool

Understand limitations and constraints from Design & Eng. team
Since we couldn’t get access to real EPB users, we sought feedback from the EPB Design Experts. They recognized our deep dive into user needs and our in-depth understanding of existing flaws, while also providing some feedback on feasibility that we could focus on.
#1 Technical & bandwidth limitations to adding dynamic gifs.
Initially we used dynamic gifs to show how the data changes during the prediction process, but were told that this was not possible due to technical and localization problems. We came up with the idea of using static but continuous diagrams to show the same process, with a larger space that fits more tailored explanation.
#2 The “Builder” pattern needs to be maintained across all other Einstein products.
“but it’s always good to think out-of-box while designing for AI 👍 ”
We proposed the "drag and drop" feature to provide a fundamentally simple and intuitive way to create models. However, since this updates the basic functional components of the Einstein series, it requires a lot of implementation costs. In the end, we adopted a version more in line with the Builder Pattern, but the Salesforce team recognized this concept as a future possibility.
User testing and results
Recruit data professionals for A/B testing
Since the EPB product is aimed at a specific group of users, we recruited participants similar to our target users to test our design. We found participants who work in the data/business analytics field and have in-depth data and business knowledge, but are less familiar with AI. Through A/B testing, we tested whether the new design solved the existing problems.
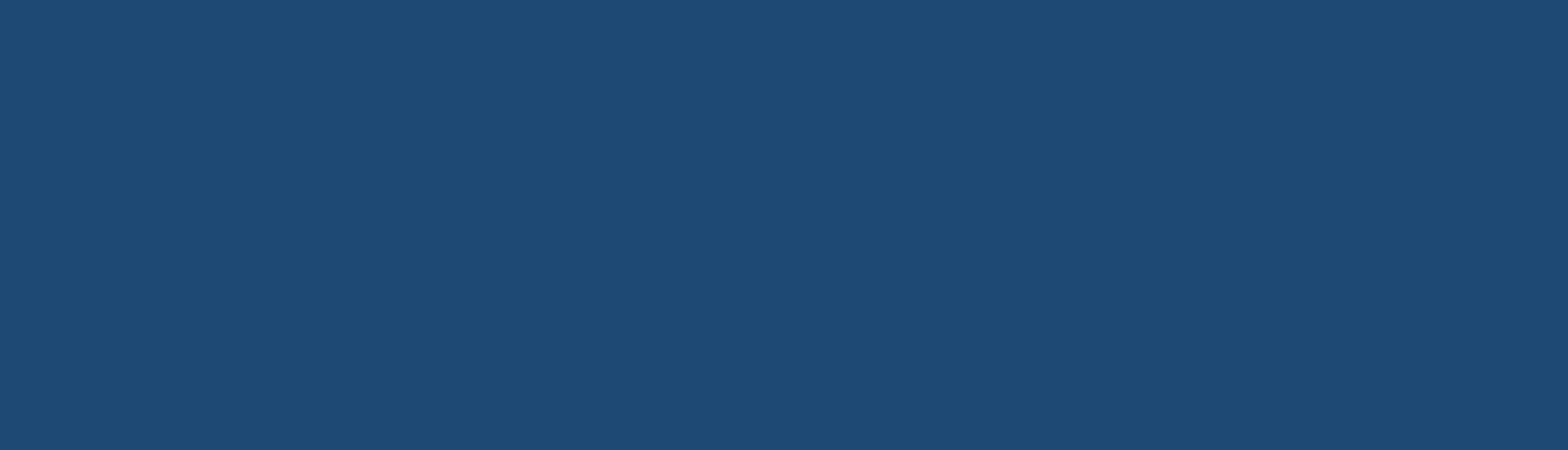

My Contribution
Bridge the gap between technology and design
As a UX designer with computer science background, I'm proud of the role I've played in this project, uniting technology and design seamlessly. By harnessing my programming background, fostering collaboration between Data Science and UX team members, and grounding innovation in real-world possibilities, I've aimed to contribute a unique blend of skills that enriched the project and delivered tangible results.
Reflection
Work with design system
During this project, we had the opportunity to work with the Salesforce Lightning Design System, and realized how it brought consistency and efficiency to our design. I also learned that the establishment and maintenance of a design system is a collaborative endeavor across design, development and other teams, and as a designer we need to actively address challenges and foster collaboration.
Embrace constraints
During this project, we learned a lot of real-world technical constraints through our discussions with the engineer team, which were very valuable feedback for us. Those are the parts that we couldn't access in our previous student passion projects. By communicating with our engineers, we learned what was technically feasible and what would be costly in terms of time, which allowed us to better evaluate and iterate our designs to achieve a balance between usability and feasibility.